CONCEPT DRIFT ISSUES IN RECOMMENDATION SYSTEM
The generic way to monitor concept drift is depicted in the following image. In other domains this change maybe called covariate shift dataset shift or nonstationarity.
My Data Drifted What S Next How To Handle Ml Model Drift In Production
Concept drift in machine learning and data mining refers to the change in the relationships between input and output data in the underlying problem over time.
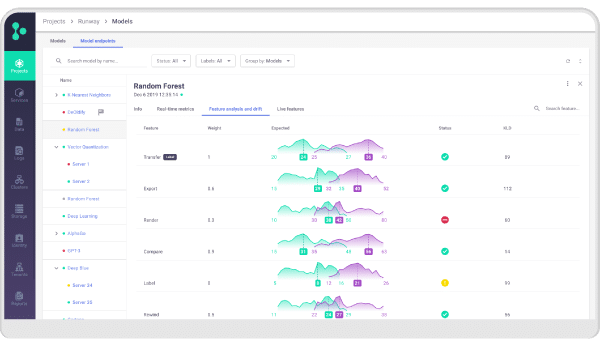
. Traditional classifiers can be easily fooled in such situations resulting in poor performances. CONCEPT DRIFT IN LEARNING PROBLEMS Relevant examples of learning problem in presence of Concept Drift includes. Concept drift monitoring system.
Recommendation systems and spam email filtering where learning task consists in predicting user preferences interests Financial market analysis where the learning task is to predict trends. So it might recommend a shipping route thats slow costly or even dangerous. Or new users and items may appear making the recommender system unable to accurately map the relationship between users and items due to the cold start problem.
1A real time recommender system. First the training data set is collected and curated then the model is trained on that. The model is continuously monitored against a golden data set which is curated by human experts.
In concept drift problem this discrepancy arises in time due to changes in the data generating process. Is referred to in the literature as concept drift 2 4 5. Concept Drift-aware cloud service APIs Recommendation CD-APIR To adapt users preference drifts and provide effective recommendation results to composite cloud system developers we propose CD-APIR.
The generic way to monitor concept drift is depicted in the following image. Nonetheless users preferences may change over time the so-called concept drifts. Considering the changes of user preferences especially the problem of concept drift the learning model for cloud service APIs recommendation faces a hidden technical debt issue ie the recommender system needs to continuously make new technical efforts to update the model to learn from new data Sculley et al 2015.
In the following sections we introduce two commonly used basic algorithms class buffers and centroid-driven. While concept drift is about changes in the target variable an output like forecasted toilet paper sales data drift occurs when there. Common concept drift domains include recommendation systems energy consumption.
Another cause of model production problems is data drift. Two key problems result from concept drift. Consequently concept drift and cold start are challenges that downgrade the recommender systems predictive.
Concept drift monitoring system. However a dataset shift can have a number of other reasons for instance sample selection bias 11 domain shift due to changes in measurements model shift due to imbalance of data 125 discrimination in decision making 71 which are out of the scope. Alzogbi 2 presented a time-aware recommender system Time-aware Collaborative Topic Regression -T-CTR that accounts for the concept-drift in.
This problemis referred to in the literature as concept drift 2 4 5. An example of Concept drift is more likely in an email recommendation system as you discover enhancements from the production data set that were not considered with the test dataset. In turn the existence of concept drift requires RSs to adapt the user modelling process to the.
First the algorithm starts making predictions that are less accurate often much less accurate. CD-APIR tracks users temporal preferences through users behavior-aware information analysis. You would want the model to evolve as quickly as possible adequately weighting recent user behavior data with historical data and show recommendations that.
Ample of a binary recommendation system the preferences may change invalidating some of the experiences stored in the buffer. The model is continuously monitored against a golden data set which is curated by human experts. Tackle concept drift early and often.
In such a case we have to address the concept drift problem and update our memory adequately. PDF On Feb 1 2019 Nayer Wanas and others published DETECTION AND HANDLING OF DIFFERENT TYPES OF CONCEPT DRIFT IN NEWS RECOMMENDATION SYSTEMS Find read and cite all the research you need on. Concept drift is healthy but it should not disqualify your efforts or heavily constrain your timeline to deployment.
First the training data set is collected and curated then the model is trained on that. Concept drift causes the output recommendations to skew from the current user interest over time and become irrelevant 6. Training new recommender models.
Singular Value Decomposition SVD and Jensen. One of the major issues facing RSs is that the users interest andor the item features themselvesmay change over time especially for data streams that online users interact with 4. Although such behavior is not usually expected in controlled environments real-world scenarios can face changes in the data such as new classes clusters and features.
Applied Sciences Free Full Text Recommender System Based On Temporal Models A Systematic Review Html
Concept Drift Deep Dive How To Build A Drift Aware Ml System Iguazio
Pdf Detection And Handling Of Different Types Of Concept Drift In News Recommendation Systems
Concept Drift Deep Dive How To Build A Drift Aware Ml System Iguazio
Concept Drift Deep Dive How To Build A Drift Aware Ml System Iguazio
Concept Drift Deep Dive How To Build A Drift Aware Ml System Iguazio
Understand And Handling Data Drift And Concept Drift
My Data Drifted What S Next How To Handle Ml Model Drift In Production
0 Response to "CONCEPT DRIFT ISSUES IN RECOMMENDATION SYSTEM"
Post a Comment